Odontology, wind turbine engineering, quantum mechanics … these are very different skill sets.
Text enhancers create skill set nodes that reflect precisely their know-how in a given language combination.
This node is the first step to matching them with projects that suit and interest them. Trust Chain™ (quality and delivery reliability) and price are the others.
Text enhancers create skill set nodes that reflect precisely their know-how in a given language combination.
This node is the first step to matching them with projects that suit and interest them. Trust Chain™ (quality and delivery reliability) and price are the others.
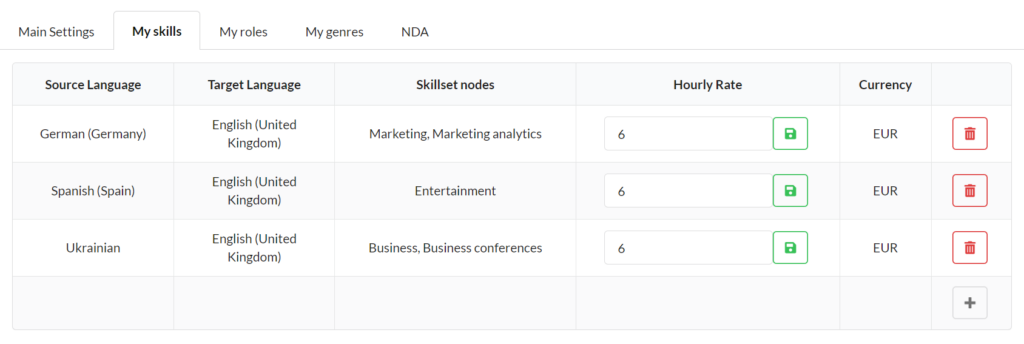
When you upload a new project, you are asked to specify which skill set nodes are relevant to your project.
Only linguists and subject matter experts who match your wishes are considered for your project.
Our platform auto-matches the skills of available community members with whatever skills are required for your project.
The result? The best possible domain expertise and linguistic quality. Every time.